Revolutionizing Cybersecurity with Machine Learning Malware Detection
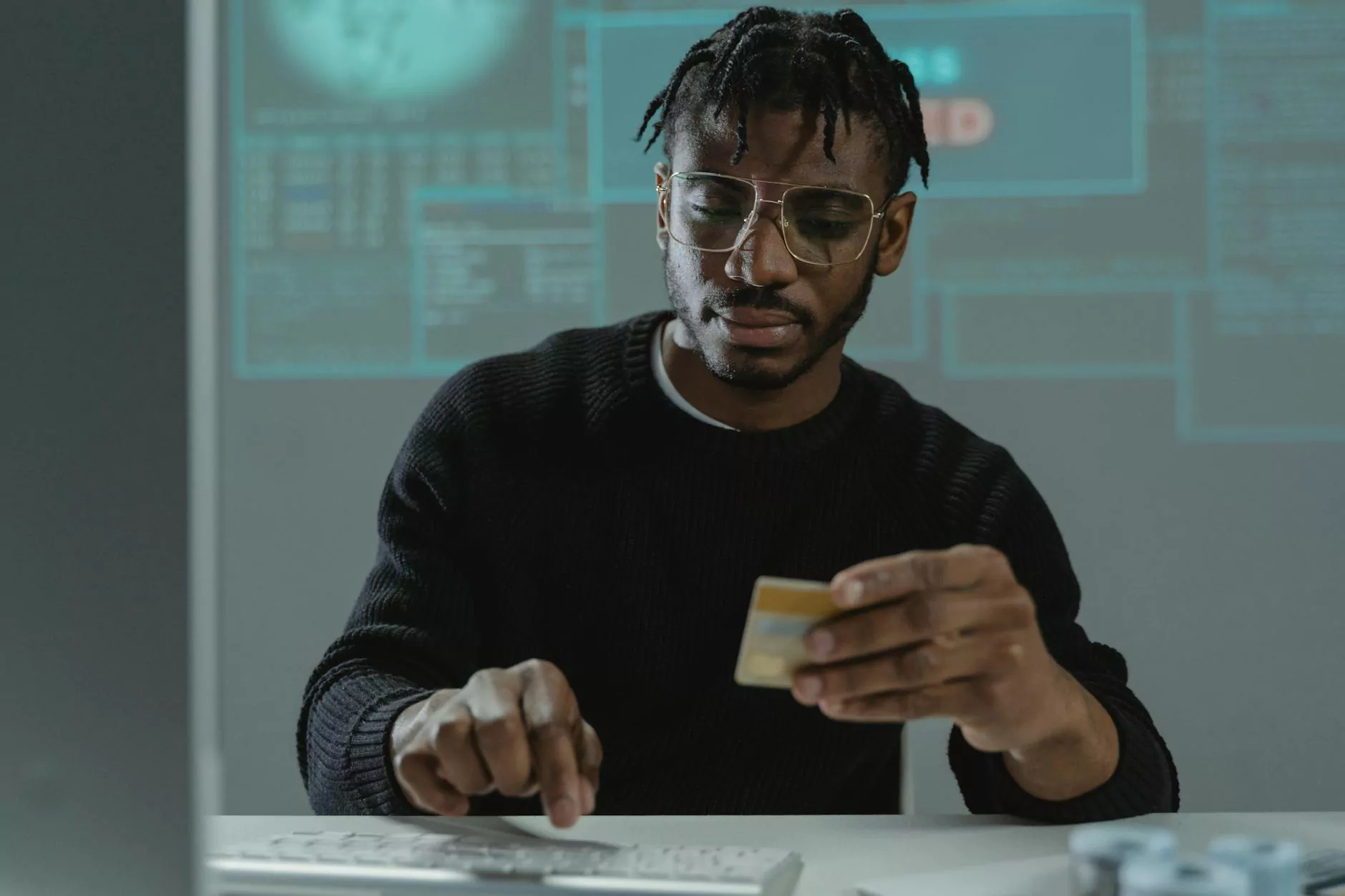
In today’s digital landscape, the threat of malicious software continues to evolve at an unprecedented pace. Traditional signature-based detection methods are increasingly insufficient against rapidly changing malware variants and zero-day attacks. As a result, organizations are turning to advanced artificial intelligence (AI) techniques, most notably machine learning malware detection, to safeguard their digital assets. This transformative approach is redefining cybersecurity effectiveness, enabling businesses to proactively identify and neutralize threats with greater accuracy and speed.
Understanding Machine Learning Malware Detection and Its Significance
Machine learning malware detection employs sophisticated algorithms that analyze vast amounts of data to identify patterns indicative of malicious activity. Unlike traditional methods that depend on known signatures, machine learning models can predict and detect new, unseen threats based on behavior and other intrinsic characteristics of malware.
How Does Machine Learning Malware Detection Work?
This technology hinges on training models with large datasets containing both malicious and benign samples. Once trained, these models can classify new files or behaviors in real-time by examining features such as:
- File attributes – size, structure, and metadata
- Behavioral patterns – network activity, system calls, process behaviors
- Code signatures – opcode sequences and code structures
- Environmental interactions – system modifications, registry changes
The model continuously learns from new data, refining its accuracy to adapt to evolving malware trends, hence providing a dynamic shield that stays ahead of cybercriminals.
The Advantages of Implementing Machine Learning Malware Detection in Business
Businesses that adopt machine learning malware detection tools benefit from numerous advantages that bolster their cybersecurity posture. These advantages are critical in maintaining operational integrity, protecting sensitive data, and ensuring customer trust.
1. Real-Time Threat Detection and Response
One of the key benefits is the ability to detect malware in real-time, significantly reducing the window for malicious activities to succeed. Machine learning models analyze behaviors as they occur, enabling instant identification and automated response to threats, thereby minimizing potential damages.
2. Detection of Unknown and Zero-Day Malware
Traditional signature-based systems are ineffective against newly emerging malware variants. In contrast, machine learning-based systems can recognize suspicious patterns and anomalies, making them highly effective against zero-day exploits that have not yet been cataloged.
3. Enhanced Accuracy and Reduced False Positives
By leveraging complex data analysis, machine learning models improve the accuracy of malware detection. This results in fewer false positives, which often cause operational disruptions and alert fatigue among cybersecurity teams.
4. Adaptive Learning and Continuous Improvement
These systems are designed to adapt over time by learning from new threats and behaviors, ensuring that security measures remain effective against the latest malware tactics. This continuous learning process makes cybersecurity defenses resilient and adaptive.
5. Cost-Effectiveness and Efficiency
Automated detection reduces the need for extensive manual oversight, thereby lowering operational costs. Businesses can reallocate resources more effectively while maintaining a high level of security.
Key Technologies Driving Machine Learning Malware Detection
The success of machine learning malware detection depends on several cutting-edge technologies working in unison:
- Supervised Learning – Utilizing labeled datasets to train models to classify files as malicious or benign.
- Unsupervised Learning – Identifying anomalies and unknown threats through clustering and pattern recognition without predefined labels.
- Deep Learning – Employing neural networks capable of analyzing complex data hierarchies for enhanced detection capabilities.
- Natural Language Processing (NLP) – Analyzing code comments, scripts, and command sequences for malicious intent.
Integrating Machine Learning Malware Detection into Business Security Architecture
Effective integration requires a robust, multi-layered approach that complements existing security measures.
Steps for Successful Implementation:
- Comprehensive Data Collection – Gather diverse data sources including network logs, endpoint data, and application activity.
- Model Training and Validation – Employ high-quality datasets to train models and validate performance before deployment.
- Deployment in Hybrid Environments – Combine machine learning tools with traditional antivirus, firewalls, and intrusion detection systems for layered security.
- Continuous Monitoring and Updating – Maintain active oversight to update models as new threats emerge.
- Employee Training & Awareness – Educate staff about emerging threats and best practices to support machine learning defenses.
Enhancing Business Security with IT Services & Computer Repair and Security Systems
For businesses like those serviced by spambrella.com, integrating advanced security systems that utilize machine learning malware detection is crucial.
- IT Services & Computer Repair: Regular updates, patches, and maintenance ensure that systems are resilient and capable of supporting sophisticated detection tools.
- Security Systems: From firewall appliances to endpoint protection, deploying AI-driven security systems helps in establishing a proactive defense framework.
Why Choose spambrella.com for Your Business's Security Needs?
spambrella.com specializes in delivering comprehensive IT security solutions tailored to your organization's unique requirements. Their expertise in integrating machine learning malware detection into security architecture helps ensure your business remains ahead of cyber threats.
By leveraging cutting-edge security systems and providing IT services & computer repair, spambrella.com guarantees that your infrastructure stays secure, efficient, and fully optimized for the modern threat landscape.
Future Trends in Machine Learning Malware Detection
The future of malware detection is poised to become even more sophisticated with innovations such as:
- Explainable AI (XAI): Enhancing transparency and trust in detection algorithms.
- Federated Learning: Collaborating across organizations without compromising data privacy.
- Integration with Threat Intelligence: Real-time sharing of malicious signatures and behavioral patterns.
- Automated Response Systems: Enabling immediate mitigation actions based on detection results.
This progress will empower businesses to create resilient, adaptive security ecosystems that stay a step ahead of cybercriminals in a continually evolving threat environment.
Conclusion: Embracing the Power of Machine Learning Malware Detection for Business Security
In conclusion, machine learning malware detection represents a paradigm shift in cybersecurity, offering superior detection capabilities, adaptability, and efficiency. Businesses that prioritize integrating these technologies into their security infrastructure gain a competitive advantage by significantly reducing risk and ensuring operational continuity. Companies like spambrella.com are at the forefront of this revolution, providing innovative IT services & computer repair combined with state-of-the-art security systems powered by machine learning.
Investing in such advanced security measures is not just a tech upgrade—it is a strategic move to safeguard your future in an increasingly digital and threat-rich environment.